WEBHASH: A Spatio-Temporal Deep Learning Approach for Detecting Social Engineering Attacks
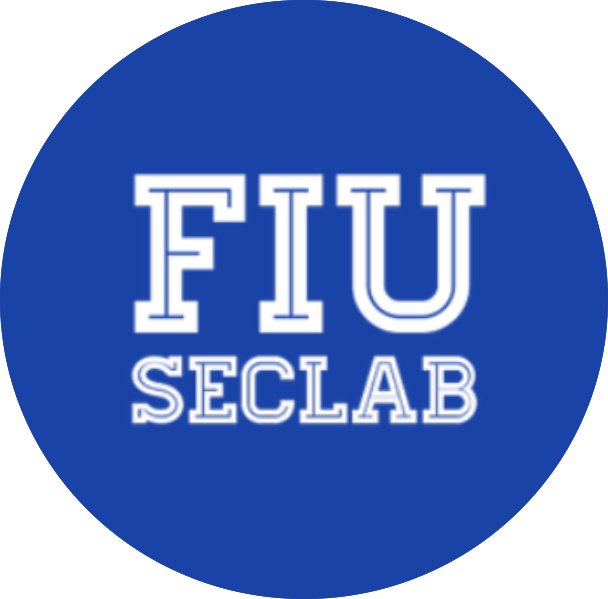
Social engineering attacks continue to remain a top security threat. The impact of these attacks is often deep and consequential. Modern social engineering attacks have evolved to deliver different classes of malicious code while collecting extensive financial and personal information. Unfortunately, current mechanisms are woefully inadequate to identify and reason about such adversarial operations, leaving organizations and end-users open to a variety of consequential attacks. The goal of this project is to design principles that will guide the development of an unsupervised approach to automatically identify temporal drifts and detect emerging trends in the social engineering attack landscape. The core insight of our research is that most of social engineering campaigns rarely change the underlying software development techniques to build their attack pages and tend to reuse specific web development patterns to generate a diverse set of attack pages. In this proposal, we develop a novel similarity hashing mechanism, called WEBHASH, which takes into account the spatio-temporal characteristics of a target website and converts them into a vector that facilitates a low-overhead attribution and similarity testing at scale. Wel take advantage of advances in machine learning to conduct unsupervised similarity testing across the vectorized data. We posit that a number of useful activities can be performed with WEBHASH. By developing low latency detection and mitigation platforms for social engineering attacks, we can better protect organizations and institutions from data breaches and reduce users’ exposure to modern social engineering attacks. WEBHASH also allows approximating the prevalence of an emerging social engineering threat or the adoption of new attack techniques across different campaigns with minimal human intervention.
Dates Active: August 2021 — January 2023
Organizations
Systems Security Lab at FIU (PI)
Microsoft M365 Security and Compliance Research (External collaborator)
Funding: Microsoft Security AI Research